AI recommendation systems are digital stalkers that never sleep. They track every move users make online – from casual scrolling to decisive clicks – building intricate profiles of preferences and behaviors. These systems combine collaborative filtering (what others like) with content-based approaches (matching past choices) to serve up personalized suggestions. Companies love it because it works: Amazon reports 35% of purchases come from recommendations. There’s much more to this clever matchmaking than meets the eye.
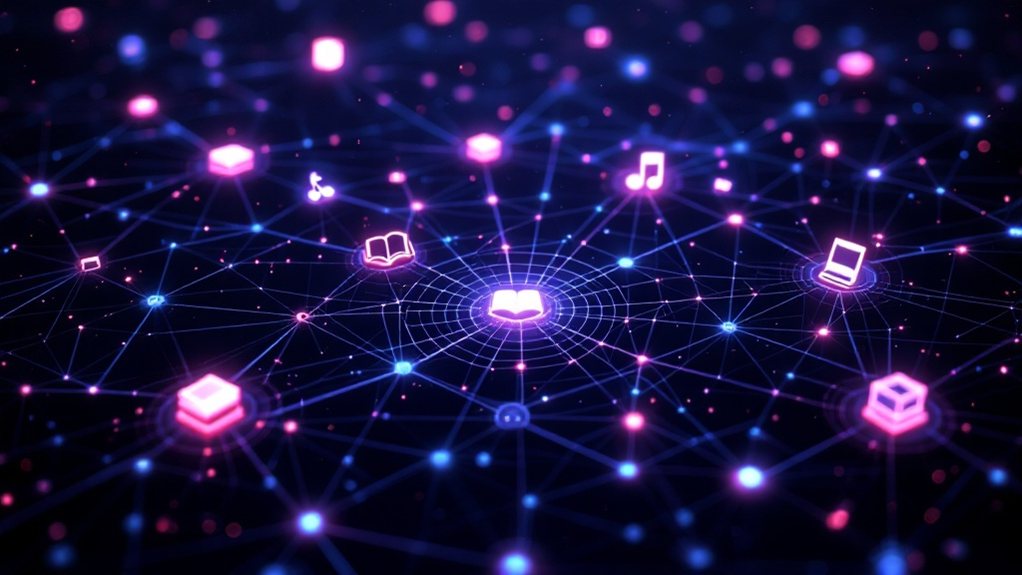
Every time you browse online, artificial intelligence is quietly studying your behavior. Like a digital stalker – but the helpful kind – AI systems track everything from your casual scrolling to your decisive clicks. These sophisticated algorithms aren’t just being nosy; they’re building an intricate profile of your preferences, helping companies serve up exactly what you’re most likely to want. And boy, does it work. Amazon has seen 35% of purchases come directly from their recommendation system.
Behind the scenes, different types of recommendation systems are constantly competing for accuracy. There’s collaborative filtering, which basically says, “Hey, you like what these other people like!” Then there’s content-based filtering, which matches item attributes to your past choices. Some systems get fancy and combine both approaches. The really show-off systems even use generative AI to create personalized content just for you. Reinforcement learning continuously adapts these recommendations based on how you respond to them.
The tech powering these recommendations is pretty mind-boggling. Neural networks crunch massive amounts of data, while natural language processing deciphers text-based content. Machine learning algorithms keep getting smarter, learning from every interaction. It’s like having a really attentive friend who never forgets anything about you – ever. Modern predictive analytics help anticipate your future choices with remarkable accuracy.
For businesses, this personalization is pure gold. Sales shoot up. Customer engagement skyrockets. Brand loyalty strengthens. It’s a win-win situation, except when it isn’t. Sometimes these systems create filter bubbles, trapping users in an echo chamber of similar content. Imagine never discovering sushi because the AI thinks you’re strictly a pizza person.
The whole system isn’t perfect, though. Getting enough quality data is a constant challenge, and new users or products throw the algorithms for a loop – the infamous “cold start” problem. Plus, these systems need to handle massive amounts of data without breaking a sweat.
But when it works, it really works. Users discover products they actually want, businesses make more money, and everyone’s happy. Well, except maybe your wallet. Because let’s face it – when recommendations are this good, resistance is futile.
Frequently Asked Questions
Can I Opt Out of AI Personalization While Still Using the Service?
Users can partially opt out of AI personalization on most platforms while still accessing core services.
The reality? It’s complicated. Many services offer toggles to limit data collection and personalization – but complete opt-out isn’t always possible.
Basic functionality often requires some level of AI processing. Platform differences make it messy too. Instagram, YouTube, Google – they all have different rules.
Perfect privacy? Good luck with that.
How Long Does It Take for AI to Learn My Preferences?
The learning speed varies dramatically. Basic preferences? Just a few interactions.
Deeper understanding takes weeks or months of regular use. AI systems start gathering data instantly, but meaningful patterns emerge over time.
Frequent usage accelerates the process – more data means better results.
But here’s the kicker: AI never stops learning. It’s constantly refining its understanding, for better or worse.
Does AI Personalization Work the Same Way Across Different Platforms?
AI personalization varies considerably across platforms. While the core principles remain similar – analyzing user behavior and adapting content – each platform has its unique quirks and data collection methods.
Netflix’s recommendation engine works differently from Spotify’s. Facebook’s ad targeting isn’t identical to Amazon’s product suggestions.
The real magic happens when these platforms sync data across channels, creating a more complete user profile. But perfect consistency? Not quite there yet.
Are My Personalized Recommendations Shared Between Different Apps and Websites?
Recommendations can be shared between apps and websites, but it’s not automatic. It depends on data sharing agreements between companies.
Some platforms actively collaborate – think Facebook and Instagram, same company, same data pool. Others? Not so much.
Privacy laws like GDPR put strict limits on data sharing, and users must typically consent first.
Cross-platform sharing also faces technical hurdles with data integration.
What Happens to My Recommendation Data if I Delete My Account?
When users delete their accounts, the impact on recommendation data gets messy.
Companies are legally required to remove personal data, but it’s not always clean or complete.
Sometimes traces remain embedded in AI models. Full deletion often requires completely retraining the systems – expensive and time-consuming.
While the visible profile disappears, the ghost of that data might linger in the algorithms.
Privacy? It’s complicated.