Racial bias in AI is a tech nightmare that keeps getting worse. These systems, trained on skewed data by mostly homogeneous development teams, consistently discriminate against minorities in healthcare, employment, and criminal justice. Facial recognition fails spectacularly with non-white individuals, while banking algorithms play favorites like a rigged casino. It’s basically Jim Crow 2.0, powered by algorithms. There’s more to this technological train wreck than meets the artificial eye.
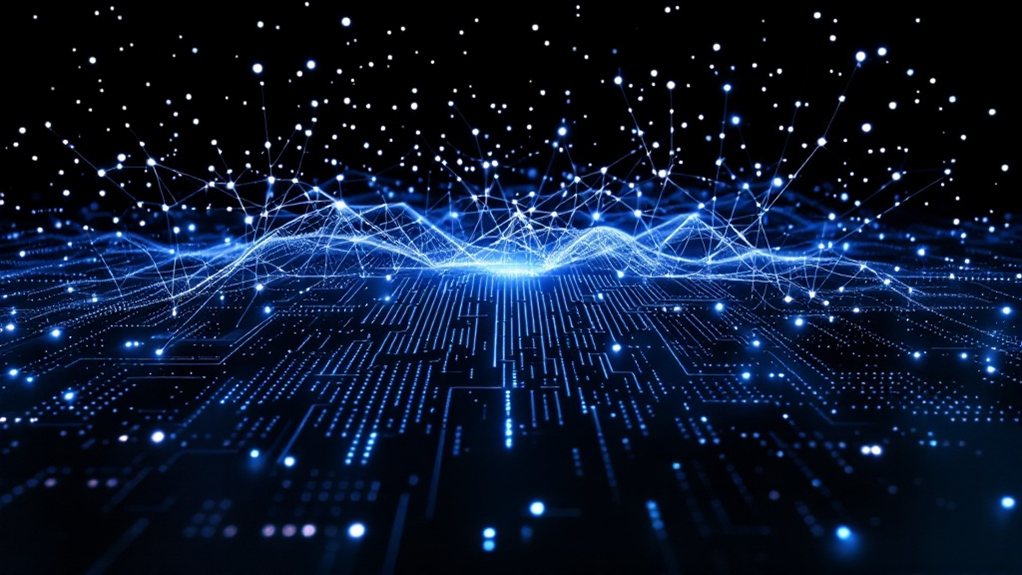
While artificial intelligence promises a future of technological advancement, its dark underbelly reveals a troubling reality: racial bias runs deep in AI systems. From biased data collection to homogeneous development teams, AI’s problems start right at the source. The tech industry’s diversity problem isn’t just about workplace politics – it’s literally being coded into our future.
The numbers don’t lie, and they’re not pretty. AI systems consistently show bias against minority groups, especially in critical areas like healthcare, employment, and criminal justice. Facial recognition? Good luck if you’re not white. Banking algorithms? They’re about as fair as a rigged carnival game. These systems are supposedly “neutral,” but they’re about as neutral as a Fox News broadcast during election season. Research shows that AI models assign lower-prestige jobs to speakers of African American English compared to those using standardized English. Word embeddings in Natural Language Processing reflect significant racial biases that mirror societal prejudices.
The impact ripples through society like a toxic wave. When AI systems discriminate against speakers of African American English or fail to recognize diverse faces, they’re not just making simple mistakes – they’re reinforcing decades-old prejudices with shiny new technology. It’s like Jim Crow got a Silicon Valley makeover. Organizations face serious legal and reputational risks when deploying biased AI systems in critical decision-making processes.
Modern AI isn’t just glitchy with diversity – it’s downloading society’s prejudices and running them through faster processors.
Statistical metrics reveal the ugly truth: AI systems consistently underperform when dealing with minority populations. The data sets? Skewed. The algorithms? Biased. The development teams? Let’s just say they’re not winning any diversity awards.
And here’s the kicker – these biases don’t just affect individual decisions; they compound over time, creating a snowball effect of discrimination that can last generations.
Some efforts are being made to address these issues through improved data collection and fairness in model design. But let’s be real – progress is moving at the speed of a turtle wearing concrete shoes.
Until the tech industry gets serious about diversity in both its workforce and its data sets, AI will continue to perpetuate the same old biases, just with more processing power. The future might be artificial, but the discrimination is very real.
Frequently Asked Questions
Can AI Systems Be Reprogrammed to Completely Eliminate Racial Bias?
Complete elimination of racial bias in AI systems remains impossible.
While reprogramming techniques like algorithmic adjustments and debiasing can reduce bias considerably, AI reflects society’s deep-rooted inequalities.
The complex nature of these systems, combined with constantly evolving biases and unrepresentative data, means some bias always sneaks through.
Progress? Yes.
Perfection? Not happening.
How Do Different Cultures Around the World Perceive AI Racial Bias?
Cultural perceptions of AI bias vary dramatically worldwide.
Western societies often view it through a racial justice lens, while Asian countries frequently focus on socioeconomic impacts.
Some African nations see it as digital colonialism 2.0.
Fascinating twist? Developing countries sometimes trust AI more than humans to be fair – despite clear evidence of bias.
Meanwhile, Nordic countries are pushing hard for transparency, because of course they are.
What Legal Frameworks Exist to Address Racial Discrimination by AI Systems?
Legal frameworks for AI discrimination are a messy patchwork. The Civil Rights Act and Fair Housing Act form the foundation, but they weren’t built for algorithms.
Colorado’s pioneering AI legislation requires businesses to conduct bias audits. The disparate impact doctrine helps – no need to prove intent, just show harmful effects.
Federal statutes like Title VII and Equal Credit Opportunity Act add protection, but huge gaps remain.
Who Should Be Held Accountable When AI Makes Racially Biased Decisions?
Multiple parties share accountability when AI makes discriminatory decisions.
AI developers bear responsibility for biased training data and model design. Companies deploying these systems must guarantee fair implementation and regular bias audits.
But regulatory bodies? They’re falling short. Current frameworks lack teeth.
The reality? It’s a complex web of responsibility, with everyone pointing fingers while bias persists. No single player can shoulder all the blame.
How Does Racial Bias in AI Affect Employment Opportunities in Tech?
Racial bias in AI hiring tools hits Black candidates hard in tech.
The systems consistently favor resumes with white-sounding names, even when qualifications are identical.
Black men face the worst discrimination, with their applications often ranked lower by AI.
It’s a tech-powered punch to diversity – these “neutral” algorithms just copy old biases, making it harder for Black professionals to break into the industry.