AI is transforming scientific research at breakneck speed. From predicting protein structures with AlphaFold to controlling nuclear fusion reactors, these smart systems are crushing traditional timelines. They’re like super-powered lab assistants, processing massive data sets in seconds and spotting patterns humans miss. GraphCast’s weather forecasting and GNoME’s materials science discoveries prove it: months of research now take days. The future of science just got a whole lot more interesting.
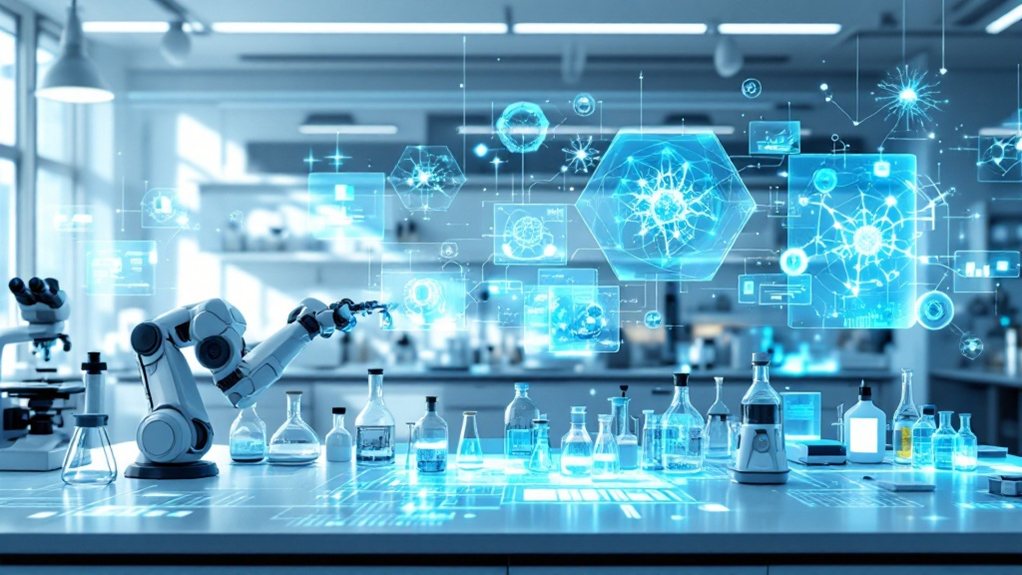
While scientists have long relied on traditional methods of research and experimentation, artificial intelligence is revolutionizing the way discoveries are made. Gone are the days of endless trial and error. AI now optimizes experiments before they even begin, predicting obstacles and suggesting improvements through sophisticated simulations. It’s like having a super-smart lab assistant who never needs coffee breaks. The limited research funding makes AI’s cost-saving capabilities particularly valuable to scientists.
AI transforms scientific discovery from guesswork to guided precision, becoming the tireless lab partner researchers always dreamed of having.
The real game-changer? AI’s ability to crunch massive amounts of data. While humans might miss subtle patterns buried in mountains of research, AI spots them instantly. It’s transforming everything from weather forecasting to protein structure prediction. Take AlphaFold, for instance – it’s solving protein structures faster than scientists can say “molecular biology.” And let’s not forget GraphCast, making weather forecasters look like amateur fortune tellers. Modern AI tools still struggle with visual data processing when interpreting complex plots and diagrams.
Scientists aren’t just getting results – they’re getting them faster. AI handles the grunt work, from literature reviews to code writing, freeing up researchers to focus on the big questions. It’s particularly impressive in fields like materials science, where tools like GNoME are discovering new materials that could revolutionize solar cells and batteries. Nuclear fusion research? AI’s there too, helping control plasma in reactors. Finally, clean energy might not be just a pipe dream. Similar to its role in predictive analytics for healthcare, AI’s ability to anticipate outcomes is transforming scientific research.
The impact on scientific productivity is undeniable. Research that once took months now takes days. Complex experiments become manageable. Data analysis becomes less about number-crunching and more about understanding implications.
Sure, AI has its limitations – it can process information like nobody’s business, but generating genuine insights? That’s still largely human territory.
But here’s the kicker: AI is uncovering patterns and connections that humans never spotted before. It’s generating novel hypotheses and predicting complex phenomena with stunning accuracy. Through tools like Deep Research, scientists are finding new connections in existing literature, leading to breakthrough discoveries.
The future of science isn’t just about artificial intelligence – it’s about intelligent collaboration between human creativity and machine precision.
Frequently Asked Questions
How Much Training Data Is Typically Needed for Scientific AI Models?
The amount of training data needed varies dramatically by model type and complexity. Supervised learning demands more data than unsupervised – often thousands or millions of labeled examples.
Simple models might work with hundreds of samples, while deep learning networks can require millions. Data quality matters more than quantity, though.
Smart techniques like transfer learning and data augmentation can reduce these requirements substantially. No one-size-fits-all answer here.
Can AI Completely Replace Human Scientists in Laboratory Research?
AI can’t fully replace human scientists – period.
While it’s great at automating routine tasks and crunching data, AI lacks the human touch where it matters most.
No contextual understanding. No true innovation. No eureka moments.
Scientists aren’t going anywhere. AI is just a fancy tool in the lab, not the brains behind breakthroughs.
Humans still need to interpret results, design experiments, and make complex decisions.
What Programming Languages Are Most Commonly Used in Scientific AI Applications?
Python dominates scientific AI, hands down. Its massive libraries like TensorFlow and Keras make it the go-to choice.
R brings serious statistical firepower, especially with data visualization.
MATLAB’s still hanging around for numerical computing.
Julia’s the new kid – blazing fast with big datasets.
C++ handles the heavy lifting when speed matters most.
JavaScript? It’s keeping AI alive in web applications.
How Do Scientists Validate the Accuracy of Ai-Generated Research Findings?
Scientists employ multiple validation methods to verify AI findings. They run analyses repeatedly to check consistency, manually sample data to confirm accuracy, and use cross-validation techniques comparing AI outputs with human-verified datasets.
NLP tools help analyze patterns and themes, while transparency in AI platforms lets researchers track processes. Issues like “hallucination” require human verification, especially in critical fields like healthcare.
Tools like SymGen speed up validation by linking outputs to data sources.
What Are the Current Limitations of AI in Analyzing Complex Scientific Data?
AI hits some serious roadblocks when tackling complex scientific data.
The infamous “black box” problem makes it tough to understand how AI reaches conclusions. Data quality issues and inherent biases can skew results completely.
Plus, AI struggles with creative leaps and challenging established theories. While great at crunching numbers, it often misses vital context and lacks the nuanced understanding that human scientists bring to the table.