AI bias is real, and it’s a huge problem. Machine learning systems consistently discriminate against minorities and underrepresented groups, leading to unfair treatment in everything from healthcare to hiring. These systems learn from historical data packed with human prejudices, resulting in skewed outcomes that hurt real people. While tech companies scramble to fix the issue with better training data and audits, the road to truly unbiased AI remains a complex journey worth exploring.
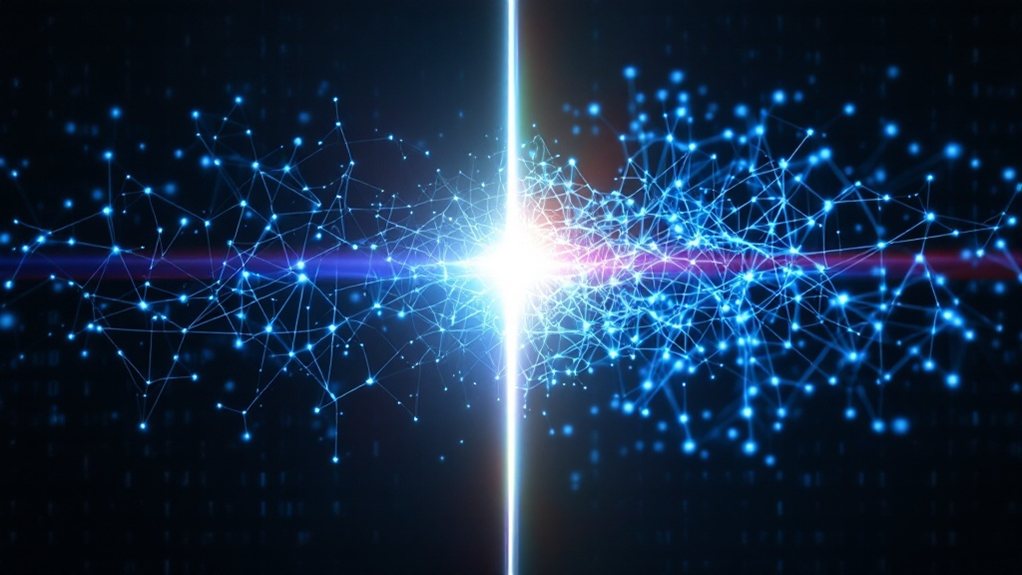
When it comes to artificial intelligence, bias isn’t just a buzzword – it’s a serious problem affecting millions of people. AI systems are learning from our messy human history, complete with all its prejudices and unfair practices. And boy, are they learning those lessons well. From facial recognition systems that can’t properly identify people of color to healthcare algorithms that shortchange minority patients, the evidence is everywhere.
The problem runs deep. Historical data? Biased. Training samples? Often skewed toward majority groups. Even the way we label data comes with its own set of prejudices. Language models show covert racial bias when processing African American English dialects. It’s like teaching a child using only books from the 1950s and expecting them to understand modern society. Spoiler alert: it doesn’t work great.
Training AI with biased historical data is like teaching modern values using only outdated textbooks – the result is predictably flawed.
Just look at what’s happening in the real world. The COMPAS tool used in criminal justice? Yeah, it turned out to be biased against Black defendants. Job recommendation systems? They’re practically playing favorites based on gender. Speech recognition technology shows higher error rates for black voices compared to white ones. Even those fun AI avatar apps can’t help but perpetuate stereotypes. It would be almost funny if it weren’t so serious.
The consequences aren’t just theoretical – they’re hitting people where it hurts. Minorities face worse healthcare outcomes because AI can’t properly diagnose their conditions. Qualified candidates get passed over for jobs because an algorithm decided they don’t “fit the profile.” And companies? They’re learning the hard way that biased AI can lead to expensive lawsuits. AI-powered diagnostic tools are particularly troubling, showing less accuracy for skin cancer detection in people with darker skin tones.
There’s hope, though. Organizations like NIST are working on standards to measure and detect bias. Companies are starting to use more diverse training data and regular auditing processes. Some cities, like San Francisco, are even banning certain AI applications until they can prove they’re fair.
It’s a start, but let’s be real – we’ve got a long way to go before AI truly treats everyone equally. After all, algorithms are only as unbiased as the humans who create them. And humans? Well, we’re still working on that part ourselves.
Frequently Asked Questions
Can Human Bias Be Completely Eliminated From AI Systems?
Completely eliminating human bias from AI systems is practically impossible.
These systems learn from human-generated data, which inherently contains societal biases and prejudices. Even with rigorous data preprocessing and diverse development teams, some biases slip through.
It’s like trying to remove all germs from a hospital – you can reduce them considerably, but total elimination? Dream on.
The goal is mitigation, not perfection.
How Do Companies Test AI Algorithms for Potential Discrimination?
Companies employ multiple testing layers to catch discriminatory AI behavior.
They run bias audits, checking if their algorithms treat different groups fairly. Data sampling helps spot demographic gaps. Statistical tests measure outcome disparities across populations.
Many use specialized bias detection tools and back-testing to compare model versions. Regular monitoring is essential – because biases can sneak in when you least expect them.
What Role Do Diversity Initiatives Play in Developing Fair AI?
Diversity initiatives play an essential role in catching bias before it becomes baked into AI systems.
Diverse teams spot problems others miss – it’s just common sense. They bring different perspectives, experiences, and cultural insights to development. From data collection to testing, having varied viewpoints helps create fairer AI.
Plus, diverse teams better understand the needs of different user groups. No single perspective can catch everything.
Should AI Algorithms Be Regulated by Government Oversight Bodies?
Government oversight of AI algorithms isn’t just necessary – it’s essential.
Complex AI systems affect everything from healthcare decisions to criminal sentencing, and their impact is too significant to go unchecked.
While regulation faces challenges keeping pace with rapid technological advancement, frameworks like the EU’s AI Act prove it’s possible.
Dedicated regulatory bodies can enforce standards, guarantee transparency, and protect against algorithmic bias.
The stakes are simply too high for a hands-off approach.
How Can Users Identify if an AI System Is Discriminating?
Users can spot AI discrimination by examining outcome patterns across different groups. Unequal results for race, gender, or age? Red flag.
Statistical tools reveal bias through disparate impact analysis and correlation checks. Watch for proxy variables too – seemingly neutral factors like zip codes often mask discrimination.
Testing with different demographic inputs helps expose unfair treatment. Real-world impacts tell the true story.